I’ve written about the relationship (or, rather, the lack of one) between language teachers and language teaching research before. I’m talking about the kind of research that is primarily of the ‘what-works’ variety, since that is likely to be of most relevance to teachers. It’s the kind of research that asks questions like: can correction be beneficial to language learners? Or: can spaced repetition be helpful in vocabulary acquisition? Whether teachers find this relevant or not, there is ample evidence that the vast majority rarely look at it (Borg, 2009).
See here, for example, for a discussion of calls from academic researchers for more dialogue between researchers and teachers. The desire, on the part of researchers, for teachers to engage more (or even a little) with research, continues to grow, as shown by two examples. The first is the development of TESOLgraphics, which aims to make research ‘easy to read and understand to ESL, EFL, EAP, ESP, ESOL, EAL, TEFL teachers’ by producing infographic summaries. The second is a proposed special issue of the journal ‘System’ devoted to ‘the nexus of research and practice in and for language teacher education’ and hopes to find ways of promoting more teacher engagement with research. Will either of these initiatives have much impact? I doubt it, and to explain why, I need to take you on a little detour.
The map and the territory
Riffing off an ultra-short story by Jorge Luis Borges (‘On Exactitude in Science’, 1946), the corpus linguist Michael Stubbs (2013) wrote a piece entitled ‘Of Exactitude in Linguistics’, which marked his professional retirement. In it, he described a world where
the craft of Descriptive Linguistics attained such Perfection that the Transcription of a single short Conversation covered the floor of an entire University seminar room, and the Transcription of a Representative Sample of a single Text-Type covered the floor area of a small department to a depth of several feet. In the course of time, especially after the development of narrow phonetic transcription with intonational and proxemic annotation, even these extensive Records were found somehow wanting, and with the advent of fully automatic voice-to-orthography transcription, the weight of the resulting Text Collections threatened severe structural damage to University buildings.
As with all humour, there’s more than a grain of truth behind this Borgesian fantasy. These jokes pick up on what is known as the Richardson Effect, named after a British mathematician who noted that the length of the coastline of Great Britain varies according to the size of the units that are used to measure it – the smaller the unit, the longer the coastline. But at what point does increasing exactitude cease to tell us anything of value?
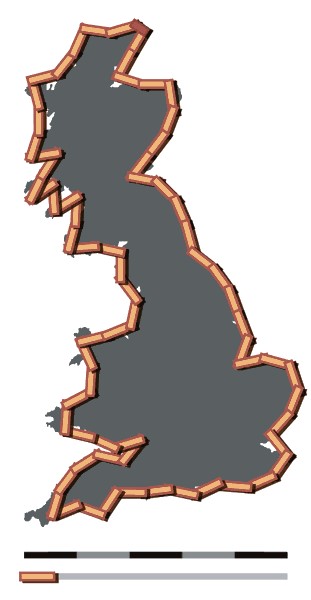
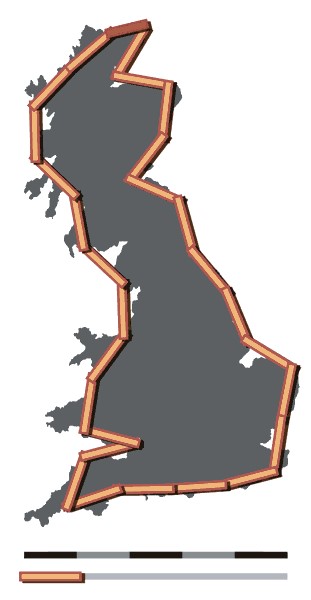
Both Borges and Lewis Fry Richardson almost certainly knew Lewis Carroll’s novel ‘Sylvie and Bruno Concluded’ (1893) which features a map that has the scale of a mile to a mile. This extraordinarily accurate map is, however, never used, since it is too large to spread out. The cost of increasing exactitude is practical usefulness.
The map of language
Language is rather like a coastline when it comes to drilling down in order to capture its features with smaller and smaller units of measurement. Before very long, you are forced into making decisions about the variety of the language and the contexts of use that you are studying. Precisely what kind of English are you measuring? At some point, you get down to the level of idiolect, but idiolects can be broken down further as they vary depending on the contexts of use. The trouble, of course, is that idiolects tell us little that is of value about the much broader ‘language’ that you set out to measure in the first place. The linguistic map obscures the linguistic terrain.
In ultra close-up, we can no longer distinguish one named language from another just by using linguistic criteria (Makoni & Pennycook, 20077:1). Extending this logic further, it makes little sense to even talk about named languages like English, to talk about first or second languages, about native speakers or about language errors. The close-up view requires us to redefine the thing – language – that we set out to define and describe. English is no longer a fixed and largely territorial system owned by native-speakers, but a dynamic, complex, social, deterritorialized practice owned by its users (May, 2013; Meier, 2017; Li Wei, 2018). In this view, both the purpose and the consequence of describing language in this way is to get away from the social injustice of native-speaker norms, of accentism, and linguistic prejudice.
A load of Ballungs
Language is a fuzzy and context-dependent concept. It is ‘too multifaceted to be measured on a single metric without loss of meaning, and must be represented by a matrix of indices or by several different measures depending on which goals and values are at play’ (Tal, 2020). In the philosophy of measurement, concepts like these are known as ‘Ballung’ concepts (Cartwright & Bradburn, 2011). Much of what is studied by researchers into language learning are also ‘Ballung’ concepts. Language proficiency and language acquisition are ‘Ballung’ concepts, too. As are reading and listening skills, mediation, metacognition and motivation. Critical thinking and digital literacies … the list goes on. Research into all these areas is characterised by multiple and ever-more detailed taxonomies, as researchers struggle to define precisely what it is that they are studying. It is in the nature of most academic study that it strives towards exactitude by becoming more and more specialised in its analysis of ‘ever more particular fractions of our world’ (Pardo-Guerra, 2022: 17).
But the perspective on language of Makoni, Pennycook, Li Wei et al is not what we might call the ‘canonical view’, the preferred viewpoint of the majority of people in apprehending the reality of the outside world (Palmer, 1981). Canonical views of language are much less close-up and allow for the unproblematic differentiation of one language from another. Canonical views – whether of social constructs like language or everyday objects like teacups or birds – become canonical because they are more functional for many people for everyday purposes than less familiar perspectives. If you want to know how far it is to walk from A to B along a coastal footpath, the more approximate measure of metres is more useful than one that counts every nook and cranny in microns. Canonical views can, of course, change over time – if the purpose to which they are put changes, too.
Language teaching research
There is a clear preference in academia for quantitative, empirical research where as many variables as possible are controlled. Research into language teaching is no different. It’s not enough to ask, in general terms, about the impact on learning of correction or spaced repetition. ‘What works’ is entirely context-dependent (Al-Hoorie, et al., 2023: 278). Since all languages, language learners and language learning contexts are ‘ultimately different’ (Widdowson, 2023: 397), there’s never any end to the avenues that researchers can explore: it is a ‘self-generating academic area of inquiry’ (ibid.). So we can investigate the impact of correction on the writing (as opposed to the speaking) of a group of Spanish (as opposed to another nationality) university students (as opposed to another age group) in an online setting (as opposed to face-to-face) where the correction is delayed (as opposed to immediate) and delivered by WhatsApp (as opposed to another medium) (see, for example, Murphy et al., 2023). We could carry on playing around with the variables for as long as we like – this kind of research has already been going on for decades.
When it comes to spaced repetition, researchers need to consider the impact of different algorithms (e.g. the length of the spaces) on different kinds of learners (age, level, motivation, self-regulation, etc.) in their acquisition of different kinds of lexical items (frequency, multi-word units, etc.) and how these items are selected and grouped, the nature of this acquisition (e.g. is it for productive use or is it purely recognition?). And so on (see the work of Tatsuya Nakata, for example).
Such attempts to control the variables are a necessary part of scientific enquiry, they are part of the ‘disciplinary agenda’, but they are unlikely to be of much relevance to most teachers. Researchers need precision, but the more they attempt to ‘approximate the complexities of real life, the more unwieldy [their] theories inevitably become’ (Al-Hoorie et al., 2023). Teachers, on the other hand, are typically more interested in canonical views that can lead to general take-aways that can be easily applied in their lessons. It is only secondary research in the form of meta-analyses or literature reviews (of the kind that TESOLgraphics) that can avoid the Richardson Effect and might offer something of help to the average classroom practitioner. But this secondary research, stripped of the contextual variables, can only be fairly vague. It can only really tell us, for example, that some form of written correction or spaced repetition may be helpful to some learners in some contexts some of the time. In need of ‘substantial localization’, it has been argued that the broad-stroke generalisations are often closer to ‘pseudo-applications’ (Al-Hoorie et al., 2023) than anything that is reliably actionable. That is not to say, however, that broad-stroke generalisations are of no value at all.
Finding the right map
Henry Widdowson (e.g. 2023) has declared himself sceptical about the practical relevance of SLA research. Reading journals like ‘Studies in Second Language Acquisition’ or ‘System’, it’s hard not to agree. Attempts to increase the accessibility of research (e.g. open-access or simple summaries) may not have the desired impact since they do not do anything about ‘the tenuous link between research and practice’ (Hwang, 2023). They cannot bridge the ‘gap between two sharply contrasting kinds of knowledge’ (McIntyre, 2006).
There is an alternative: classroom-based action research carried out by teachers. One of the central ideas behind it is that teachers may benefit more from carrying out their own research than from reading someone else’s. Enthusiasm for action research has been around for a long time: it was very fashionable in the 1980s when I trained as a teacher. In the 1990s, there was a series of conferences for English language teachers called ‘Teachers Develop Teachers Research’ (see, for example, Field et al., 1997). Tirelessly promoted by people like Richard Smith, Paula Rebolledo (Smith et al., 2014) and Anne Burns, action research seems to be gaining traction. A recent British Council publication (Burns, 2023) is a fine example of what insights teachers may gain and act on with an exploratory action research approach.
References
Al-Hoorie A. H., Hiver, P., Larsen-Freeman, D. & Lowie, W. (2023) From replication to substantiation: A complexity theory perspective. Language Teaching, 56 (2): pp. 276 – 291
Borg, S. (2009) English language teachers’ conceptions of research. Applied Linguistics, 30 (3): 358 – 88
Burns, A. (Ed.) (2023) Exploratory Action Research in Thai Schools: English teachers identifying problems, taking action and assessing results. Bangkok, Thailand: British Council
Cartwright, N., Bradburn, N. M., & Fuller, J. (2016) A theory of measurement. Working Paper. Centre for Humanities Engaging Science and Society (CHESS), Durham.
Field, J., Graham, A., Griffiths, E. & Head. K. (Eds.) (1997) Teachers Develop Teachers Research 2. Whitstable, Kent: IATEFl
Hwang, H.-B. (2023) Is evidence-based L2 pedagogy achievable? The research–practice dialogue in grammar instruction. The Modern Language Journal, 2023: 1 – 22 https://onlinelibrary.wiley.com/doi/full/10.1111/modl.12864
Li Wei. (2018) Translanguaging as a Practical Theory of Language. Applied Linguistics, 39 (1): 9 – 30
Makoni, S. & Pennycook, A. (Eds.) (2007) Disinventing and Reconstituting Languages. Clevedon: Multilingual Matters
May. S. (Ed.) (2013) The multilingual turn: Implications for SLA, TESOL and Bilingual education. New York: Routledge
McIntyre, D. (2006) Bridging the gap between research and practice. Cambridge Journal of Education 35 (3): 357 – 382
Meier, G. S. (2017) The multilingual turn as a critical movement in education: assumptions, challenges and a need for reflection. Applied Linguistics Review, 8 (1): 131-161
Murphy, B., Mackay J. & Tragant, E. (2023) ‘(Ok I think I was totally wrong: new try!)’: language learning in WhatsApp through the provision of delayed corrective feedback provided during and after task performance’, The Language Learning Journal, DOI: 10.1080/09571736.2023.2223217
Palmer, S.E. et al. (1981) Canonical perspective and the perception of objects. In Longand, J. & Baddeley. A. (Eds.) Attention and Performance IX. Hillsdale, NJ: Erlbaum. pp. 135 – 151
Pardo-Guerra, J. P. (2022) The Quantified Scholar. New York: Columbia University Press
Smith, R., Connelly, T. & Rebolledo, P. (2014). Teacher research as CPD: A project with Chilean secondary school teachers. In D. Hayes (Ed.), Innovations in the continuing professional development of English language teachers (pp. 111–128). The British Council.
Tal, E. “Measurement in Science”, In The Stanford Encyclopedia of Philosophy (Fall 2020 Edition), Edward N. Zalta (Ed.), https://plato.stanford.edu/archives/fall2020/entries/measurement-science/
Widdowson, H. (2023) Webinar on the subject of English and applied linguistics. Language Teaching, 56 (3): 393 – 401