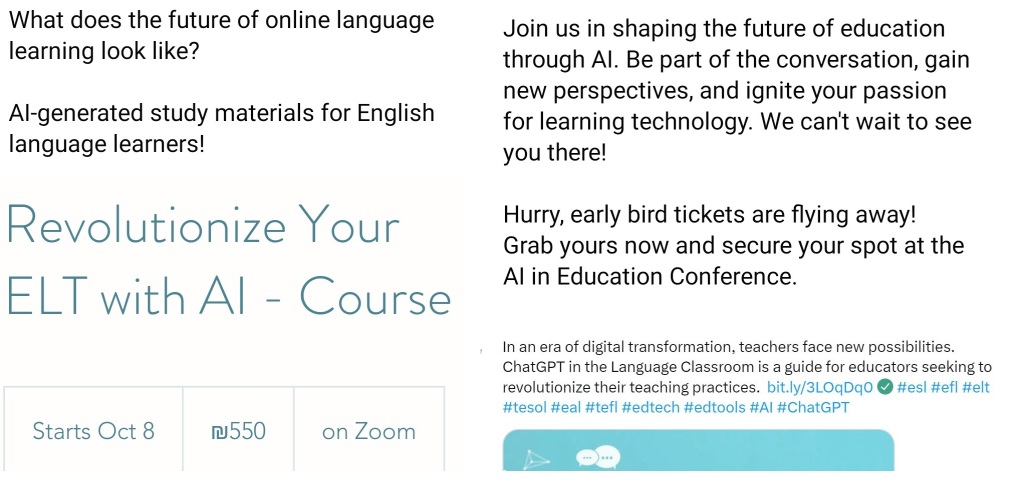
In the world of ELT teacher blogs, magazines, webinars and conferences right now, you would be hard pressed to avoid the topic of generative AI. Ten years ago, the hot topic was ‘mobile learning’. Might there be some lessons to be learnt from casting our gaze back a little more than a decade?
One of the first ELT-related conferences about mobile learning took place in Japan in 2006. Reporting on this a year later, Dudeney and Hockly (2007: 156) observed that ‘m-learning appears to be here to stay’. By 2009, Agnes Kukulska-Hulme was asking ‘will mobile learning change language learning?’ Her answer, of course, was yes, but it took a little time for the world of ELT to latch onto this next big thing (besides a few apps). Relatively quick out of the blocks was Caroline Moore with an article in the Guardian (8 March 2011) arguing for wider use of mobile learning in ELT. As is so often the case with early promoters of edtech, Caroline had a vested interest, as a consultant in digital language learning, in advancing her basic argument. This was that the technology was so ubiquitous and so rich in potential that it would be foolish not to make the most of it.
The topic gained traction with an IATEFL LT SIG webinar in December 2011, a full-day pre-conference event at the main IATEFL conference early the following year, along with a ‘Macmillan Education Mobile Learning Debate’. Suddenly, mobile learning was everywhere and, by the end of the year, it was being described as ‘the future of learning’ (Kukulska-Hulme, A., 2012). In early 2013, ELT Journal published a defining article, ‘Mobile Learning’ (Hockly, N., 2013). By this point, it wasn’t just a case of recommending teachers to try out a few apps with their learners. The article concludes by saying that ‘the future is increasingly mobile, and it behoves us to reflect this in our teaching practice’ (Hockly, 2013: 83). The rhetorical force was easier to understand than the logical connection.
It wasn’t long before mobile learning was routinely described as the ‘future of language learning’ and apps, like DuoLingo and Busuu, were said to be ‘revolutionising language learning’. Kukulska-Hulme (Kukulska-Hulme et al., 2017) contributed a chapter entitled ‘Mobile Learning Revolution’ to a handbook of technology and second language learning.
In 2017 (books take a while to produce), OUP brought out ‘Mobile Learning’ by Shaun Wilden (2017). Shaun’s book is the place to go for practical ideas: playing around with photos, using QR codes, audio / video recording and so on. The reasons for using mobile learning continue to grow (developing 21st century skills like creativity, critical thinking and digital literacy in ‘student-centred, dynamic, and motivating ways’).
Unlike Nicky Hockly’s article (2013), Shaun acknowledges that there may be downsides to mobile technology in the classroom. The major downside, as everybody who has ever been in a classroom where phones are permitted knows, is that the technology may be a bigger source of distraction than it is of engagement. Shaun offers a page about ‘acceptable use policies’ for mobile phones in classrooms, but does not let (what he describes as) ‘media scare stories’ get in the way of his enthusiasm.
There are undoubtedly countless examples of ways in which mobile phones can (and even should) be used to further language learning, although I suspect that the QR reader would struggle to make the list. The problem is that these positive examples are all we ever hear about. The topic of distraction does not even get a mention in the chapter on mobile language learning in ‘The Routledge Handbook of Language Learning and Technology’ (Stockwell, 2016). Neither does it appear in Li Li’s (2017) ‘New Technologies and Language Learning’.
Glenda Morgan (2023) has described this as ‘Success Porn in EdTech’, where success is exaggerated, failures minimized and challenges rendered to the point that they are pretty much invisible. ‘Success porn’ is a feature of conference presentations and blog posts, genres which require relentless positivity and a ‘constructive sense of hope, optimism and ambition’ (Selwyn, 2016). Edtech Kool-Aid (ibid) is also a feature of academic writing. Do a Google Scholar search for ‘mobile learning language learning’ to see what I mean. The first article that comes up is entitled ‘Positive effects of mobile learning on foreign language learning’. Skepticism is in very short supply, as it is in most research into edtech. There are a number of reasons for this, one of which (that ‘locating one’s work in the pro-edtech zeitgeist may be a strategic choice to be part of the mainstream of the field’ (Mertala et al., 2022)) will resonate with colleagues who wish to give conference presentations and write blogs for publishers. The discourse around AI is, of course, no different (see Nemorin et al., 2022).
Anyway, back to the downside of mobile learning and the ‘media scare stories’. Most language learning takes place in primary and secondary schools. According to a recent report from Common Sense (Radesky et al., 2023), US teens use their smart phones for a median of 4 ½ hours per day, checking for notifications a median of 51 times. Almost all of them (97%) use their phones at school, mostly for social media, videos or gaming. Schools have a variety of policies, and widely varying enforcement within those policies. Your country may not be quite the same as the US, but it’s probably heading that way.
Research suggests that excessive (which is to say typical) mobile phone use has a negative impact on learning outcomes, wellbeing and issues like bullying (see this brief summary of global research). This comes as no surprise to most people – the participants at the 2012 Macmillan debate were aware of these problems. The question that needs to be asked, therefore, is not whether mobile learning can assist language learning, but whether the potential gains outweigh the potential disadvantages. Is language learning a special case?
One in four countries around the world have decided to ban phones in school. A new report from UNESCO (2023) calls for a global smart phone ban in education, pointing out that there is ‘little robust research to demonstrate digital technology inherently added value to education’. The same report delves a little into generative AI, and a summary begins ‘Generative AI may not bring the kind of change in education often discussed. Whether and how AI would be used in education is an open question (Gillani et al., 2023)’ (UNESCO, 2023: 13).
The history of the marketing of edtech has always been ‘this time it’s different’. It relies on a certain number of people repeating the mantra, since the more it is repeated, the more likely it will be perceived to be true (Fazio et al., 2019): this is the illusory truth effect or the ‘Snark rule[1]’. Mobile learning changed things for the better for some learners in some contexts: claims that it was the future of, or would revolutionize, language learning have proved somewhat exaggerated. Indeed, the proliferation of badly-designed language learning apps suggests that much mobile learning reinforces the conventional past of language learning (drilling, gamified rote learning, native-speaker models, etc.) rather than leading to positive change (see Kohn, 2023). The history of edtech is a history of broken promises and unfulfilled potential and there is no good reason why generative AI will be any different.
Perhaps, then, it behoves us to be extremely sceptical about the current discourse surrounding generative AI in ELT. Like mobile technology, it may well be an extremely useful tool, but the chances that it will revolutionize language teaching are extremely slim – much like the radio, TV, audio / video recording and playback, the photocopier, the internet and VR before it. A few people will make some money for a while, but truly revolutionary change in teaching / learning will not come about through technological innovation.
References
Dudeney, G. & Hockly, N. (2007) How to Teach English with Technology. Harlow: Pearson Education
Fazio, L. K., Rand, D. G. & Pennycook, G. (2019) Repetition increases perceived truth equally for plausible and implausible statements. Psychonomic Bulletin and Review 26: 1705–1710. https://doi.org/10.3758/s13423-019-01651-4
Hockly, N. (2013) Mobile Learning. ELT Journal, 67 (1): 80 – 84
Kohn, A. (2023) How ‘Innovative’ Ed Tech Actually Reinforces Convention. Education Week, 19 September 2023.
Kukulska-Hulme, A. (2009) Will Mobile Learning Change Language Learning? reCALL, 21 (2): 157 – 165
Kukulska-Hulme, A. (2012) Mobile Learning and the Future of Learning. International HETL Review, 2: 13 – 18
Kukulska-Hulme, A., Lee, H. & Norris, L. (2017) Mobile Learning Revolution: Implications for Language Pedagogy. In Chapelle, C. A. & Sauro, S. (Eds.) The Handbook of Technology and Second Language Teaching and Learning. John Wiley & Sons
Li, L. (2017) New Technologies and Language Learning. London: Palgrave
Mertala, P., Moens, E. & Teräs, M. (2022) Highly cited educational technology journal articles: a descriptive and critical analysis, Learning, Media and Technology, DOI: 10.1080/17439884.2022.2141253
Nemorin, S., Vlachidis, A., Ayerakwa, H. M. & Andriotis, P. (2022): AI hyped? A horizon scan of discourse on artificial intelligence in education (AIED) and development, Learning, Media and Technology, DOI: 10.1080/17439884.2022.2095568
Radesky, J., Weeks, H.M., Schaller, A., Robb, M., Mann, S., and Lenhart, A. (2023) Constant Companion: A Week in the Life of a Young Person’s Smartphone Use. San Francisco, CA: Common Sense.
Selwyn, N. (2016) Minding our Language: Why Education and Technology is Full of Bullshit … and What Might be Done About it. Learning, Media and Technology, 41 (3): 437–443
Stockwell, G. (2016) Mobile Language Learning. In Farr, F. & Murray, L. (Eds.) The Routledge Handbook of Language Learning and Technology. Abingdon: Routledge. pp. 296 – 307
UNESCO (2023) Global Education Monitoring Report 2023: Technology in Education – A Tool on whose Terms?Paris: UNESCO
Wilden, S. (2017) Mobile Learning. Oxford: OUP
[1] Named after Lewis Carroll’s poem ‘The Hunting of the Snark’ in which the Bellman cries ‘I have said it thrice: What I tell you three times is true.’